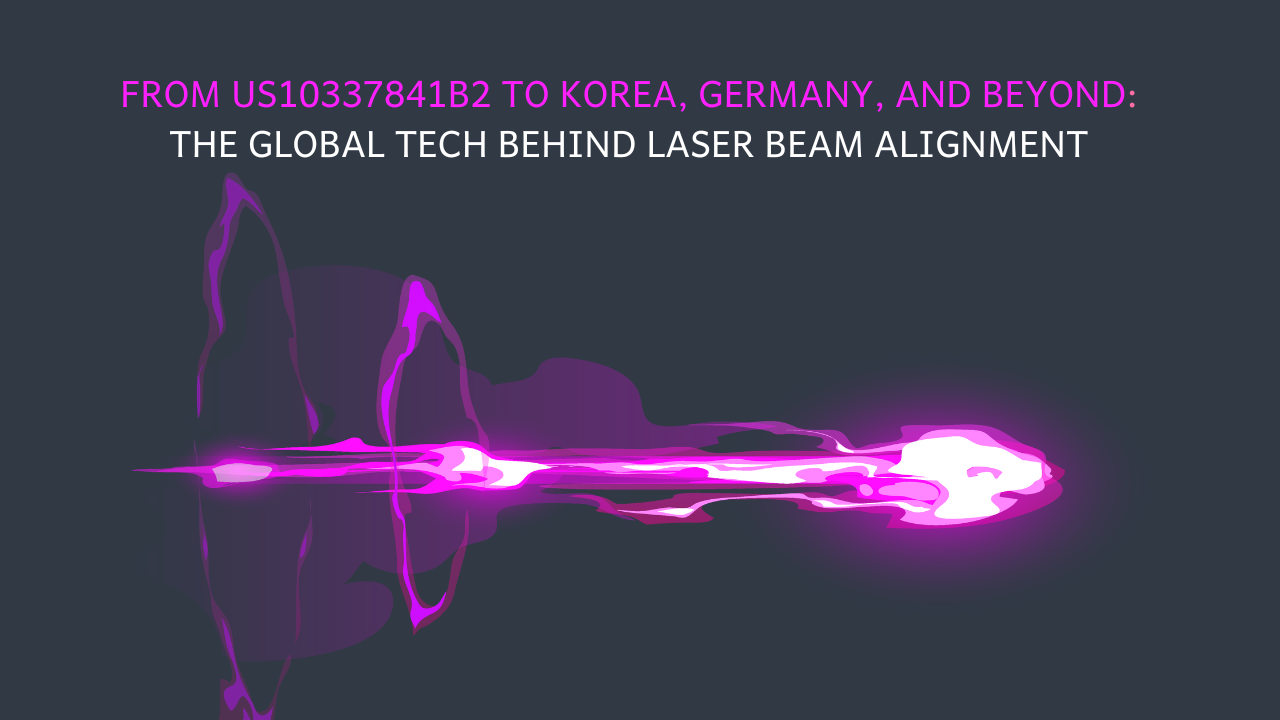
From US10337841B2 to Korea, Germany, and Beyond: The Global Tech Behind Laser Beam Alignment
In recent years, we’ve seen high-energy laser systems move from DARPA prototypes to battlefield interceptors. Israel’s Iron Beam has reportedly shot down rockets and drones